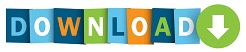
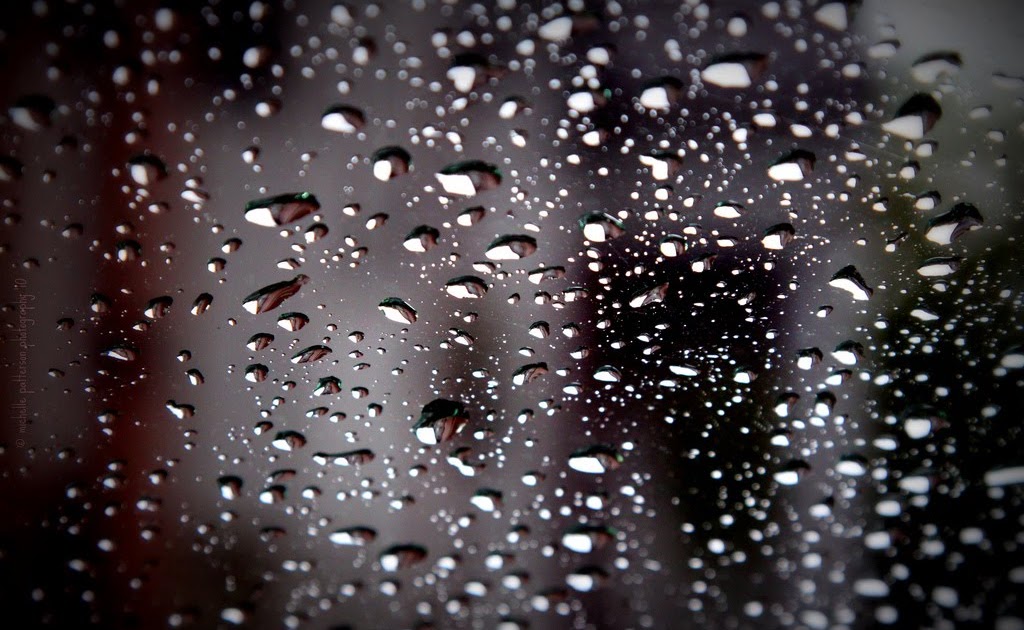
In: Proceedings of the IEEE/CVF Conference on Computer Vision and Pattern Recognition. A model-driven deep neural network for single image rain removal. Multi-scale progressive fusion network for single image deraining. Density-aware single image de-raining using a multi-stream dense network. Deep joint rain detection and removal from a single image. Removing rain from single images via a deep detail network.
Raindrop photos software#
A software platform for vision-based UAV autonomous landing guidance based on markers estimation. Attentive generative adversarial network for raindrop removal from a single image. Comprehensive experimental results demonstrate the significant superiority of the proposed models over state-of-the-art methods. To better model the heterogeneously distributed raindrops, a multi-scale dense residual block is designed to construct the hierarchical rainy-to-clean image translation network. Specifically, a two stage network is designed with the first stage generating the soft masks, which helps learn a context-enhanced representation in the second stage. With the aim of learning a more powerful raindrop removal model, we propose learning a soft mask map explicitly for mitigating the imbalanced distribution problem. None of the existing raindrop removal networks consider this underlying issue, thus resulting in the learned representation biased towards modeling raindrop regions while paying less attention to the important contextual regions. In this paper, we revisited the rainy-to-clean translation networks and identified the issue of imbalanced distribution between raindrops and varied background scenes. Despite the great success achieved by convolutional neural networks in addressing the raindrop removal problem, the still relatively blurry results call for better problem formulations and network architectures.
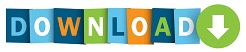